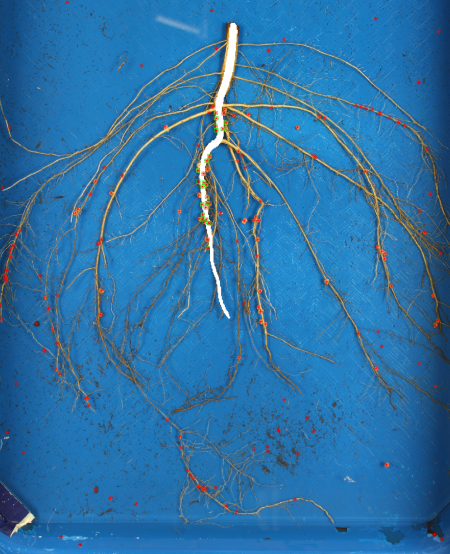
Crop producers know that yield depends on a lot that occurs underground. This is true across all plants, including soybeans. Plant roots interact with a complex microbiome in the soil, but in soybean one of the most important interactions of roots is with a bacterium that is in nodules growing on the roots. These bacteria convert atmospheric nitrogen into ammonia, which is vital to a soybean’s development.
Too few nodules? Yield is affected. Too many? Yield is affected. There is a sweet spot when it comes to soybean root nodules, and therefore researchers have an interest to study plant-bacteria relationships, and eventually, plant breeders are interested to develop varieties with that optimal amount of root nodulation. This part of phenotyping has been historically labor-intensive. But an interdisciplinary team of researchers at Iowa State University have recently developed a faster and less intensive way to monitor and quantify root nodes on a soybean plant.
“Nodules allow soybean plants to fix nitrogen from the atmosphere into an ammonia form of nitrogen so it is a useable form for the plant, and as breeders and researchers we really care about how much protein is in our seed,” said Clayton Carley, Iowa State graduate student focusing on plant breeding, genetics and genomics working in the research group of Dr. A.K. ‘Danny’ Singh, professor of agronomy and Bayer Chair in Soybean Breeding. “And if we are able to change, manipulate or investigate how these nodules are forming and developing, then potentially we can improve the plant growth and development and potentially protein acquisition in our seeds and plants overall.”
Carley recently was an author of a published paper on the development of a system to more easily calculate the nodules on a soybean plant using machine learning and a fully automated Soybean Nodule Acquisition Pipeline (SNAP).
“The reason we developed SNAP was because we wanted to explore how many nodules there were and try to quantify their sizes with which roots they are on,” Carley said.
It started with human identification of nodules by examining photographs, and Carley noticed that he could give the same photograph to the same undergrad student and there would be up to a 17% difference from the first viewing to the second.
“That meant that it was people not the plants that were changing the number,” Carley said. “That was when we started doing a lot of collaboration with engineers.”
Carley worked with engineering research scientist Dr. Zaki Jubery, who was the co-lead author, to develop SNAP. They trained SNAP through an algorithm that can look for nodules, identify them quickly, and give a count. SNAP also is able to identify the taproot of the plant, so it can differentiate between taproot nodules and non-taproot as well. SNAP will consistently give the same results and takes only about a minute and a half to do the entire pipeline on a photo, saving anywhere from six to twenty times the amount of time it would take manually.
“All of that comes with information about each nodule too, so that when I look at my dataset, I can see how big that nodule is and where it is in the picture, so I can start to calculate area and over time I can start to look at how these nodules are growing in size during various growth stages for each genotype,” Carley said. “We are looking for the best genotypes, and then figuring out which genes are causing that optimum nodule count and then we can select those genes and advance those in our breeding pipeline in order to help improve yield and explore protein acquisition too.
“We can evaluate genotypes a lot faster, traditionally the limiting factor was how fast we could evaluate the roots,” Carley said. “Now with SNAP, we are no longer limited by how fast we can evaluate it because we can use this system and don’t have a drain on human resources and now are only limited by how fast we can dig and grow the roots.”
This project builds on an earlier work co-led by Dr. Singh and Dr. Baskar Ganapathysubramanian and the Soynomics team, where Dr. Jubery and Dr. Kevin Falk (a previous Ph.D. student in the Singh group, now working at Corteva AgriSciences) developed a computer vision and machine learning-based phenotyping tool ARIAv2 that enables root trait extraction.
“This work is an excellent example of interdisciplinary work in the Soynomics groups at Iowa State University that is working to provide new tools in the hands of researchers and farmers to solve complex phenotyping problems while solving pertinent science questions,” said Dr. Singh.
Clayton is now using SNAP to disentangle relationships between soybean varieties, nodule count and size, and nodule placement.
The research support for this work came from the Iowa Soybean Research Center, Iowa Soybean Association, R.F. Baker Center for Plant Breeding, Plant Sciences Institute, Bayer Chair in Soybean Breeding, and more recently on continued projects through the National Science Foundation and USDA-NIFA.